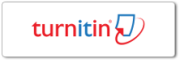
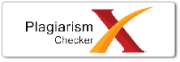
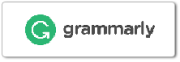
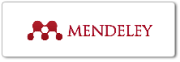
Baskoro (2010). Implementasi Algoritma KMeans Menggunakan Data Penyewaan Alat
Berat Untuk Melakukan Estimasi Nilai
Outcome, Fakultas Ilmu Komputer,
Universitas Pembangunan Nasional
â€Veteranâ€, Jakarta.
Besdek (1981). Euclidean. dlm. Eko Prasetyo.
Data Mining : Konsep dan Aplikasi
Menggunakan Matlab, Yogyakarta: ANDI.
Budi Santoso (2007), Data Mining Teknik
Pemanfaatan Data untuk Keperluan Bisnis.
Yogyakarta : Graha Ilmu.
Cary Liniker Simbolon, Nilamsari Kusumastuti
dan Beni Irawan (2013), Buletin Ilmiah Mat.
Stat. Dan Terapannya (Bimaster). Clustering
Lulusan Mahasiswa Matematika FMIPA
UNTAN Pontianak Menggunakan Algoritma
Fuzzy C-Means. 2 . 21-26
Carlos Ordonez (2004). Industry/Government
Track Poster. Programming The K-Means
Clustering Algorithm in SQL. 1-6
Davies, and Paul Beynon (2004). Database
Systems Third Edition. Palgrave Macmillan.
Eko Prasetyo (2012). Data Mining : Konsep dan
Aplikasi Menggunakan Matlab, Edisi 1,
Yogyakarta : Andi.178-201.
Elmasri, Ramez and Shamkant B. Navathe,
(2000), “Fundamentals of Database
Systems. Third Editionâ€, Addison Wesley
Publishing Company, New York.
Han, Jiawei, Kamber dan Micheline (2006),
Data Mining Concepts and Techniques
Second Edition.
Iko Pramudiono (2003). Pengantar Data Mining
: Menambang Permata Pengetahuan di
Gunung Data. Kuliah Umum
IlmuKomputer.Com. 1-4
Mahendiran et.al, (2012), Implementation of KMeans Clustering in Cloud Computing
Environment.
Michael W. Trosset (2008). Department of
Statistics. Representing Clusters : K-Means
Clustering, Self-Organizing Maps, and
Multidimensional Scaling. 8. 1-18
Narwati (2006), Pengelompokan Mahasiswa
Menggunakan Algoritma K-Means. 1-7
Tan, P; Steinbach; & Kumar, (2006), V,
Introduction to Data Mining. Pearson
Education.
Tapas Kanungo et. all (2002). Ieee Transactions
On Pattern Analysis And Machine
Intelligence. An Efficientk-Means Clustering
Algorithm: Analysis and Implementation. 24.
-12
U.M. Fayyad, G. Piatetsky-Shapiro, P. Smyth, and
R. Uthurusamy, (1996), Advances in
Knowledge Discovery and Data Mining,
AAAI/MIT Press.
Tedy Rismawan dan Sri Kusumadewi (2008).
Seminar Nasional Aplikasi Teknologi
Informasi 2008 (SNATI 2008). Aplikasi KMeans Untuk Pengelompokan Mahasiswa
Berdasarkan Body Mass Index (BMI) dan
Ukuran Kerangka. E43-E47
Vance Faber (1994). Los Alamos Science.
Clustering and The Continuous K-Means
Algorithm. 22. 1-7
Witten, Ian H. and Frank, Eibe, (2005), Data
Mining Practical Machine Learning Tools
and Techniques, Second Edition.
Widyawati, (2010), Perbandingan Clustering
Based On Frequent Word Sequence (CFWS)
Dan K-Means Untuk Pengelompokan
Dokumen Berbahasa Indonesia. Fakultas
Pendidikan Matematika Dan Ilmu
Pengetahuan Alam, Universitas Pendidikan
Indonesia, Bandung,
Yiu-Ming Cheung (2003). Pattern Recognition
Letters. K-Means: A New Generalized KMeans Clustering Algorithm. 24. 1-11
Yudi Agusta (2007), Sistem dan Informatika. KMeans Penerapan, Permasalahan dan
Metode Terkait. 3. 47-60.
INFORMATIKA
Journal URL: https://jurnal.ulb.ac.id/index.php/informatika
Journal DOI: 10.36987/informatika
P-ISSN: 2303-2863
E-ISSN: 2615-1855
Alamat Redaksi :
Fakultas Sains dan Teknologi, Universitas Labuhanbatu
Gedung Fakultas Sains dan Teknologi,
Jalan Sisingamangaraja No.126 A KM 3.5 Aek Tapa, Bakaran Batu, Rantau Sel., Kabupaten Labuhan Batu, Sumatera Utara 21418